Generative AI has taken the spotlight ever since the launch of ChatGPT—an AI model that efficiently conjures up contextual responses to user queries in seconds.
However, seasoned technologists understand that it’s not magic; it’s the result of extensive work with Large Language Models (LLMs) and vast amounts of data, employing Machine Learning (ML) to comprehend and respond to natural language prompts.
Organizations are intrigued by the many potentials of Generative AI and are seeking ways to embed the technology into their operations. To pioneer success in this realm, organizations need a strategic approach that guides them to select the most suitable models, refine them to align with unique business contexts, mitigate risks, and drive holistic success.
Choosing the Right LLM Model
Each organization holds a unique blend of industry insights, operational nuances, and a wealth of historical data such as customer interactions, sales records, financial metrics market trends, and more – elements that are too intricate to be captured by a one-size-fits-all model. With a multitude of LLMs prevalent in the market today, organizations face the challenge of selecting the most suitable model for their unique business needs.
Many organizations initially turn to “off-the-shelf” models, commonly referred to as foundation models. While these models provide a range of capabilities, they often prove inadequate in addressing the unique requirements of individual businesses, resulting in suboptimal performance and a diminished impact on customer experiences. The trade-off becomes evident: the speed and simplicity gained come at the expense of control and customization.
Conversely, organizations have the option to construct their custom models, exclusively trained with their proprietary data, providing unparalleled control. However, the training of such models necessitates access to extensive datasets and specialized infrastructure, posing significant hurdles for many companies. The associated costs of building and maintaining these models render it an impractical choice for most organizations, especially those in the early stages of AI adoption.
Considering the constraints outlined above, fine-tuning becomes the linchpin for augmenting the value and impact of AI models— paving the way for unprecedented success. Through fine-tuning, organizations can harness and enhance their existing domain data, striking the equilibrium between customization and efficiency. This process introduces increased flexibility and adaptability for model selection and improvement, allowing adjustments based on performance and outcomes. Looking ahead, organizations that fine-tune their models in the context of their business ecosystem will be poised to maximize returns on investment and drive sustained value.
Conquering Challenges in Fine-Tuning LLMs
Fine-tuning, despite its apparent simplicity, poses several challenges that organizations must handle thoughtfully. These include the complexities of data preparation, ensuring data privacy and security, managing latency, determining the right model size, and optimizing infrastructure costs.
For example, when the existing data is inadequate or of poor quality, the primary emphasis should be on laying a solid data foundation before delving into Generative AI implementation.
Additionally, it becomes imperative to implement rigorous data handling and security protocols to safeguard sensitive customer information and ensure compliance with privacy regulations. Diligent planning, including the meticulous selection of the right hardware based on processing power, memory, and storage requisites, as well as choosing optimal scaling options, is also pivotal for cost and efficiency optimization.
Another challenge pertains to addressing risks, biases, and ethical considerations. Navigating these considerations is paramount in the fine-tuning process, ensuring that models are developed and optimized with fairness, transparency, and unbiased outcomes in mind. By embedding ethical principles into the fine-tuning process, organizations can forge a path toward responsible AI practices, contributing to a technology landscape that preempts deceptive outcomes and prioritizes equitable results.
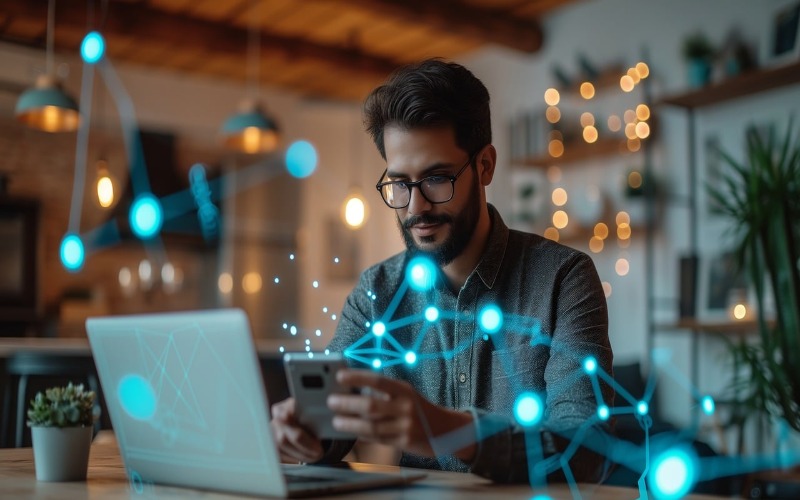
Building a Framework
Throughout the stages of model selection, fine-tuning, and risk mitigation, organizations need a powerhouse framework that serves as a guiding force to steer them through their Generative AI integration journey. This framework must lead organizations through critical considerations such as architectural principles, technology stack, responsible design, and other pivotal factors at key developmental stages of LLM-based applications. The overarching objective of this framework should be to empower organizations to build solutions tailored to their unique business context.
It should also equip them with the appropriate solution approaches and model orchestration and facilitate effective deployment through pre-built scripts. Ultimately, the adoption of such a framework must go beyond facilitating integration; it must act as a catalyst for fueling innovative use cases.
Despite being in the early stages of deployment, organizations are gearing up for the adoption of Generative AI models, recognizing their potential to adeptly address a spectrum of challenges and elevate team productivity. Three broad use cases gaining momentum in today’s dynamic business landscape are:
Conversational bots with self-serving capabilities:
Leveraging Generative AI models, businesses can develop chatbots and Intelligent Control Towers imbued with conversational capabilities. These chatbots can effortlessly navigate, condense, and retrieve information from extensive files, irrespective of document type, delivering contextual responses in seconds and offering the best self-service capabilities. This strategic implementation can effectively alleviate the workload on subject matter experts engaged in repetitive tasks.
Real-time, personalized transaction experience:
The collaboration of Generative AI and Machine Learning models holds the potential to not only enhance personalization but also offer instant, real-time access to information and heighten overall engagement experiences for customers, suppliers, employees, and other stakeholders. This integration can be utilized to build and deploy use cases in domains like service desks, field services, supply chain visibility, marketing, and eCommerce, enhancing transaction experiences across all digital touchpoints.
Intelligent ecosystem & decision autonomy:
The full potential of Generative AI will materialize through seamless integration with advanced technologies like voice assistants, speech-to-text, and robotic process automation. This integration will propel real-time, intelligent decision-making, endowing Generative AI with the capability to automate comprehensive transactions for customers and employees alike and amplify operational efficiency. Its prowess in driving informed, autonomous decisions based on parameters like value, risk, and likelihood will help businesses fortify and refine their processes according to their preferences.
Conclusion
The implementation of Generative AI is a multi-faceted journey that requires careful consideration at every step. A robust strategy, adept at model selection and overcoming fine-tuning challenges while adhering to ethical principles, can be the bedrock for propelling impactful use cases and driving tangible results. This strategy will fuel the successful implementation of diverse solutions and guarantee the responsible deployment of Generative AI, charting a conscientious course for businesses seeking to harness its transformative potential.