Public safety and the justice system are two growing areas of interest for citizens and police in the United States. Between political and racial tensions, unrest has been on the rise in drastic ways this year. Specifically, government officials and civil rights activists have turned a spotlight on police violence. More than ever, people are questioning how helpful or hurtful predictive policing is in today’s society.
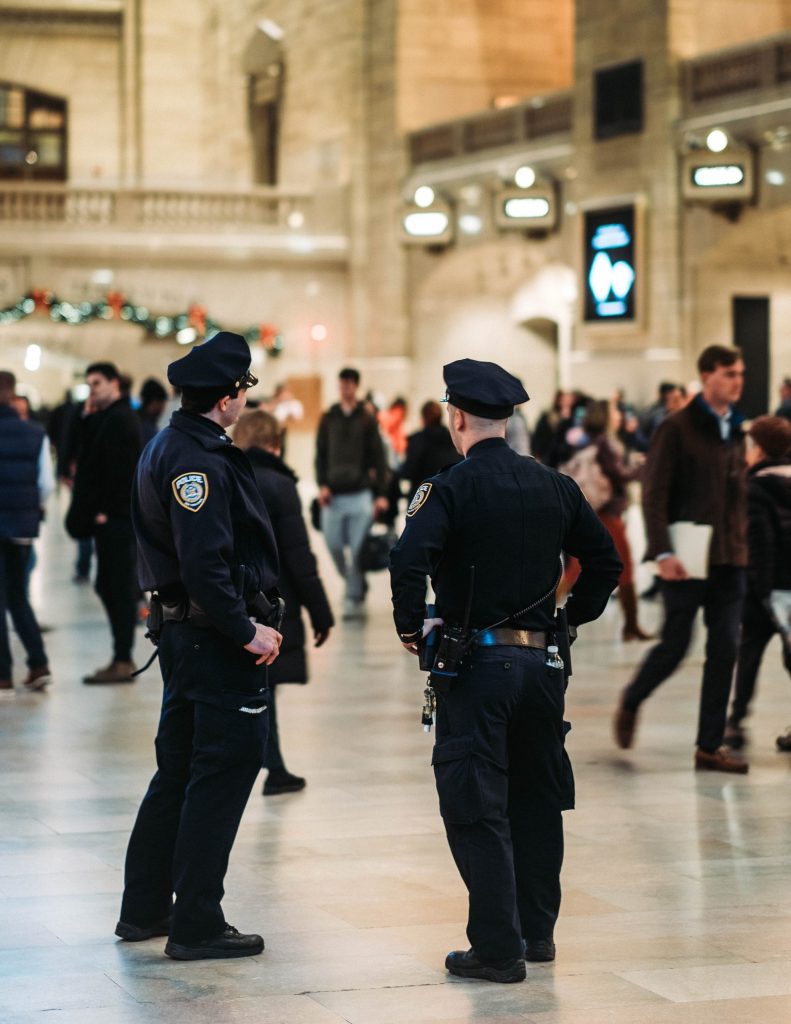
Predictive policing involves the use of high-tech systems and algorithms to determine where crime might occur. Police departments use geographical information alongside historical data, demographics, populations and economic conditions to get results.
This technology could be helpful if police can use it to reduce crime by stopping it before it even happens. For instance, if police use mapping to find hotspots for crime, they can station deputies in those areas and prevent or intercept illegal activity.
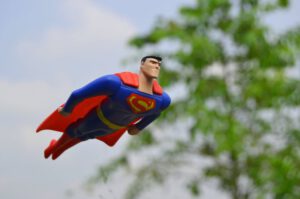
From New Jersey to Chicago, police precincts use this technology. In theory, it helps reduce crime and improve overall public safety. However, justice activists and organizations have brought up concerns about racial profiling and biases. The algorithms and data collection stir issues of historical stereotypes and future violence.
Biases in the System
Part of the reason for the growing backlash toward predictive policing is the possibility of biases on both ends of the algorithms — inputs and outcomes. Police base much of the information that goes into the predictive system on historical data.
However, activists posit that previous knowledge does not indicate where future crime will occur — or who will commit it.
For instance, PredPol is a leader in the tech field for crime prediction. The company, though, faced backlash in the past due to predictive gang violence tracking and mapping. The system was supposed to reduce gang-related violence and crime, but civil rights organizations pointed out the potential for biases.
If whoever programs these algorithms inputs racial biases — consciously or not — the system will contribute to more prejudice. In PredPol’s case, activists feared the predictions would disproportionately single out Black and Latino individuals.
Even a bias-free system can still unfairly target people of color. California, for instance, found “rampant” inaccuracies in a gang database, CalGang.
Activists point to both these instances as inaccurate crime prediction, stating that past data does not influence future crime trends and can lead to profiling.
Effectiveness
The goal of predictive policing is to reduce crime. You’ll notice technology plays an invaluable role in this process — the algorithms for crime prediction attempt to contribute to this innovation. It builds upon previous public safety steps. For instance, security case studies show the impact of physical security measures. Predictive policing seeks to be impactful in similar ways and assist in determining future security actions.
However, the backlash against this technology has been robust, and there’s a lack of evidence that prediction technology effectively reduces crime.
In central Florida, one police department that used predictive technology found itself in hot water. The algorithms with this tech aimed to predict which citizens would commit crimes in the future. However, after an investigation, the evidence showed no reduction in crime. Instead, 21 families claimed police deputies harassed them — despite no evidence of illegal activity.
Ideally, the algorithms would take a colorblind approach and not consider race in their predictions. However, a ProPublica study found that, in a Florida sample, these systems labeled 23.5% of white defendants as high-risk, compared to 44.9% of Black defendants.
Thus, the effectiveness of reducing crime is insignificant. However, there’s ample evidence of racial profiling and biases.
Alternatives to Crime Reduction
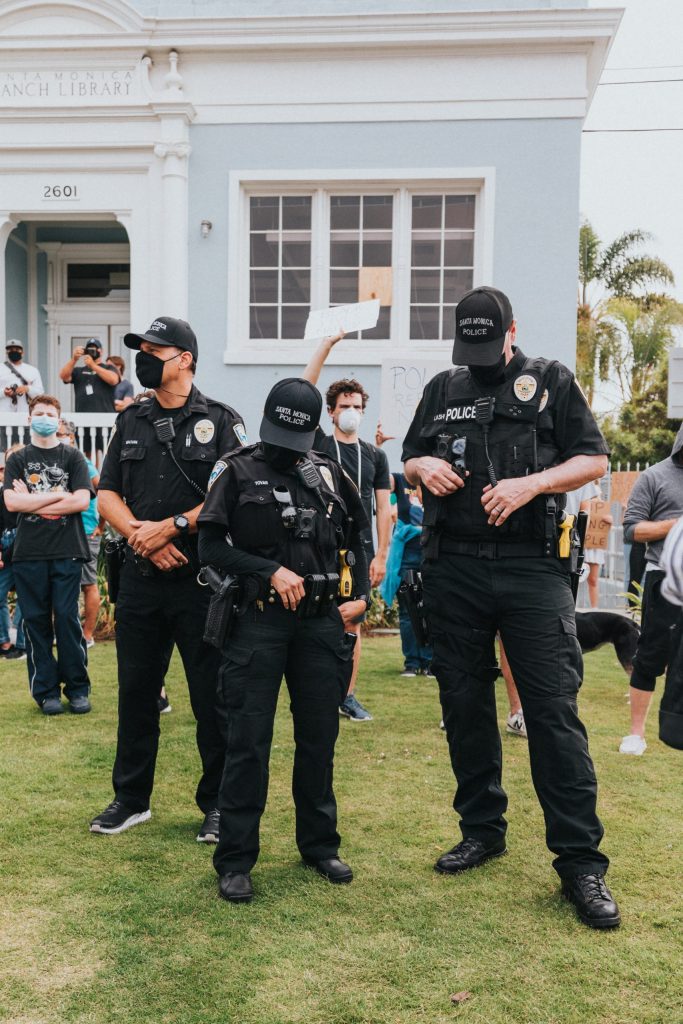
With the evidence pointing toward problems with predictive policing, it’s time to look for alternative solutions. Some police departments turn to biometrics such as facial recognition and identification as a reliable system.
However, like predictive data, face ID comes with racial biases and dilemmas as well.
Instead, tech experts are using the Internet of Things (IoT) for solutions. For instance, streetlights with infrared, internet-connected cameras can transmit video and data in real time.
Then, police can respond accordingly to the situation. Some IoT tech can even detect weapon deployment and alert law enforcement in an instant.
This tech can increase response time and, in turn, lower the amount of crime that occurs. Still, residents and police departments want to prevent crime before it happens. Increasing resources will be an invaluable step toward accomplishing this. Instead of looking at historical crime data, police can assess why crime is occurring.
If there is a lack of resources for education, economic opportunities and jobs, health care, housing, rehabilitation and accessibility, crime will likely increase correspondingly. Taking proactive steps to increase funding for these areas and other social developments can reduce crime on small and large scales.
Progressing Toward the Goal
Ultimately, reducing crime is the objective. Finding the pathway there, though, has many obstacles. Predictive policing has thus far proven to do more harm than good. Using technology for public safety must follow other routes to be more effective — through things like IoT innovations and social developments.