As artificial intelligence (AI) and machine learning (ML) technologies rapidly evolve, they are reshaping the landscape of regulatory compliance. Traditional compliance frameworks struggle to keep pace with the speed of technological advancements, posing a challenge for industries governed by strict regulations, such as finance, healthcare, and data protection. The adoption of AI and ML not only enhances the accuracy and efficiency of compliance monitoring but also introduces complex questions about accountability and ethical use. In this transformative era, nearly 60% of organizations are now leveraging AI to assist with compliance activities, recognizing its potential to automate and refine compliance processes (source: Gartner).
Open-source technologies are increasingly integral to this evolution, offering transparent, community-driven solutions that accelerate the deployment of robust AI/ML systems. By using open-source platforms, companies can reduce development costs, increase innovation, and ensure greater adaptability to changing regulations. Tools like TensorFlow and Apache Kafka enable developers to build and scale applications that can manage vast quantities of data and complex compliance requirements, making open-source technology a cornerstone of modern compliance strategies. This collaborative approach not only democratizes access to cutting-edge technologies but also fosters a more inclusive environment for regulatory technology development.
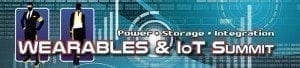
The Role of AI/ML in Enhancing Regulatory Compliance
AI/ML technologies are revolutionizing the approach to regulatory compliance by providing tools that can predict potential non-compliance issues before they become problematic. For instance, predictive analytics can forecast risky behaviors or anomalies in financial transactions, which are crucial for sectors like banking and securities trading. Automated monitoring systems further enhance this by continually scanning data streams for deviations from regulatory norms. These AI-driven systems not only reduce the burden of manual oversight but also enhance accuracy and response times, leading to more effective compliance management. Companies such as JPMorgan have implemented machine learning to analyze legal documents and extract essential data, thus ensuring quicker and more accurate compliance reviews.
One notable example is the use of AI by Danske Bank to detect suspicious transactions as part of its anti-money laundering efforts. The bank employs AI to analyze millions of transactions in real time, significantly improving the detection rates of potential money laundering activities compared to traditional methods. This AI implementation demonstrates how technology can scale compliance operations, improve accuracy, and reduce human error.
Such advancements underscore the transformative potential of AI/ML in compliance roles. By automating complex and voluminous tasks, AI not only frees up human resources to focus on more strategic issues but also sets a new standard in maintaining regulatory compliance. As these technologies become more refined, their adoption across different industries is expected to rise, further embedding AI at the core of regulatory strategies. For further reading on AI in compliance, resources like the IBM Watson compliance solutions provide in-depth insights into practical applications and benefits (source: IBM Watson).
Open-Source Tools and Their Impact on Compliance
Open-source tools are pivotal in the deployment of AI/ML technologies in regulatory compliance, with platforms like TensorFlow, Scikit-learn, and Apache Kafka leading the way. TensorFlow offers a comprehensive ecosystem of tools, libraries, and community resources that allow researchers and developers to build and deploy machine learning applications efficiently. Similarly, Scikit-learn provides simple and efficient tools for data mining and data analysis, which are accessible to everybody and reusable in various contexts. Apache Kafka, a distributed streaming platform, enables firms to process and analyze data in real time, which is critical for maintaining compliance in dynamic regulatory environments.
The transparency of open-source software is one of its greatest assets in regulatory compliance. With source code that is accessible to everyone, it’s easier to ensure that the software adheres to compliance standards without hidden functionalities. This transparency fosters trust, a crucial component in regulatory contexts, especially when handling sensitive or critical data. Furthermore, the community-driven nature of open-source projects enhances the reliability and security of the software, as a large community of developers continually tests, refines, and validates the code.
Adaptability is another significant advantage. Open-source tools can be customized to meet specific regulatory requirements of different industries. This flexibility allows companies to tailor solutions that are fine-tuned to their compliance needs, providing an edge over proprietary software that often comes as a one-size-fits-all solution. This adaptability not only makes open-source tools cost-effective but also ensures they can evolve with changing regulations, making them indispensable in modern compliance frameworks.
Challenges and Future Directions
Integrating AI/ML and open-source technologies into compliance frameworks presents several technical and ethical challenges. On the technical front, ensuring data quality and managing data privacy are paramount, especially under regulations such as GDPR. AI systems require vast amounts of data to learn and make decisions, which can raise concerns about the security and confidentiality of the data used. Ethically, there is the risk of bias in AI algorithms, which can lead to unfair or discriminatory outcomes if not carefully managed. The open-source nature of many AI tools can also complicate accountability, as it can be difficult to pinpoint responsibility for errors or compliance failures in a broadly distributed development environment.
Looking ahead, the increasing adoption of AI in regulatory compliance is expected to continue, with emerging trends focusing on greater integration of real-time data processing and advanced predictive analytics. Technologies such as blockchain might play a critical role in enhancing transparency and security, particularly in industries like finance and healthcare where data integrity is crucial. The use of natural language processing (NLP) to automate and enhance the monitoring of compliance with ever-changing regulations is also on the rise.
These technological advancements promise a more dynamic regulatory compliance landscape. As AI and machine learning technologies become more sophisticated, their integration into compliance frameworks is likely to become more seamless, offering more proactive and less reactive compliance solutions. However, it will be crucial for organizations to stay vigilant about the ethical implications of these technologies, ensuring that compliance tools not only adhere to regulatory standards but also uphold the principles of fairness and transparency.
Pioneering Compliance in the Digital Age
As organizations continue to integrate AI/ML and open-source technologies into their regulatory frameworks, the emphasis on innovation in compliance strategies becomes increasingly pivotal. This integration not only addresses current regulatory demands but also sets the groundwork for future-proofing compliance practices. The key to achieving this lies in fostering a culture of continuous learning and adaptation within organizations. By actively participating in open-source communities and collaborating on the development of AI-driven compliance tools, companies can tap into a wealth of knowledge and stay at the forefront of technology trends.
Moreover, as regulatory environments inevitably evolve, the flexibility provided by AI/ML technologies enables organizations to swiftly adapt to new regulations. This adaptability will be crucial for maintaining compliance without sacrificing operational efficiency. In embracing these technological advancements, companies must also prioritize the ethical dimensions of AI, ensuring that these powerful tools enhance fairness and transparency in compliance procedures. In doing so, businesses not only meet the stringent demands of today’s regulatory frameworks but also contribute to shaping the ethical use of AI in the broader industry, leading the charge toward a more accountable and technologically adept future.